When is a statistic valid?
Validity in statistics refers to whether a measurement tool or method actually measures what you want to measure. A statistic is valid when it actually measures what you want to measure and is not just an approximation or a distortion of it.
There are different types of validity, such as internal validity and external validity. Internal validity refers to whether the results of a study can actually be attributed to the influence of the independent variable and not to other factors. External validity, on the other hand, refers to whether the results of a study can be generalized to other populations, units, or settings.
There are several ways to support or question the validity of a statistic. However, this depends on the specific circumstances and variables under consideration. It is important to consider the specific requirements of the study and the variables under consideration to appropriately support or question the validity of a statistic.
A statistic is valid if it has both high internal and high external validity. This means that it actually measures what it claims to measure.
What is meant by validity in statistics?
In statistics, validity refers to the accuracy and reliability of a measurement procedure or analysis. A measurement procedure or analysis is valid if it actually measures what it purports to measure.
There are several types of validity, which can be defined differently depending on the specific context and variables under consideration. Examples of types of validity include construct validity, content-related validity, and criterion-related validity.
Validity is an important factor to consider when collecting or analyzing data. A measurement procedure or analysis that is not valid may lead to incorrect conclusions and thus be of little use.
What does validity say?
Validity indicates how accurate and reliable a measurement procedure or analysis is. A measurement procedure or analysis is valid if it actually measures what it intends to measure.
It is important to note that the validity of a measurement procedure or analysis can be defined differently depending on the specific context and variables under consideration.
Interne Validität
It is important to consider both aspects of validity when conducting or analyzing experimental studies. High internal validity indicates that the results of the study are likely due to the influence of the independent variable, rather than other factors. High external validity, on the other hand, indicates that the results of the study are generalizable to other populations, units, or settings. It is possible for a study to have high internal validity but low external validity, or vice versa.
Internal validity in detail
Internal validity refers to whether the results of a study can actually be attributed to the influence of the independent variable and not to other factors. It indicates whether the results of the study are actually due to the influence of the independent variable and not to other factors. High internal validity indicates that the results of the study are actually due to the influence of the independent variable and not to other factors. Low internal validity, on the other hand, indicates that the results of the study may not be due to the influence of the independent variable and that other factors may play a role.
There are several ways to improve the internal validity of a study, such as using control groups, including multiple measurements, and excluding possible bias factors.
How is internal validity established?
Internal validity is not usually calculated directly, but rather supported or challenged by the design and conduct of the study. There are several ways to improve the internal validity of a study, such as using control groups, including multiple measurements, and excluding potential bias factors.
To support the internal validity of a study, one should ensure that the independent variable was indeed manipulated and that the sample was randomly selected. One should also make sure that possible bias factors that could affect the results of the study have been excluded or controlled for.
It is important to note that there is no absolute “right” or “wrong” way to support or question the internal validity of a study. Rather, it depends on the specific circumstances and variables under consideration as to which methods are best suited to support or challenge the internal validity of a study. However, there are some general requirements that apply to supporting internal validity, such as:
- Use of control groups: The use of control groups can eliminate potential bias factors that could influence the results of the study.
- Inclusion of multiple measurements: By including multiple measurements, one can ensure that the results of the study are not due to chance or measurement error.
- Exclusion of bias factors: by excluding bias factors (e.g., by randomizing the sample), one can ensure that the results of the study are indeed due to the influence of the independent variable and not to other factors.
There are other ways to support or challenge the internal validity of a study. It is important to consider the specific requirements of the study and the variables under consideration in order to
External validity
It is important to consider both aspects of validity when conducting or analyzing experimental studies. High internal validity indicates that the results of the study are likely due to the influence of the independent variable, rather than other factors. High external validity, on the other hand, indicates that the results of the study are generalizable to other populations, units, or settings. It is possible for a study to have high internal validity but low external validity, or vice versa.
Internal validity in detail
Internal validity refers to whether the results of a study can actually be attributed to the influence of the independent variable and not to other factors. It indicates whether the results of the study are actually due to the influence of the independent variable and not to other factors. High internal validity indicates that the results of the study are actually due to the influence of the independent variable and not to other factors. Low internal validity, on the other hand, indicates that the results of the study may not be due to the influence of the independent variable and that other factors may play a role.
There are several ways to improve the internal validity of a study, such as using control groups, including multiple measurements, and excluding possible bias factors.
How is internal validity established?
There are several ways to improve the external validity of a study, such as using representative samples and accounting for possible bias factors.
To support the external validity of a study, one should ensure that the sample is representative of the population under consideration and that possible bias factors that could influence the results of the study are taken into account.
It is important to note that there is no absolute “right” or “wrong” way to support or question the external validity of a study. Rather, which methods are best suited to support or challenge the external validity of a study depends on the specific circumstances and variables under consideration. However, there are some general requirements that apply to supporting external validity, such as:
- Use of representative samples: By using representative samples, one can ensure that the results of the study are generalizable to other populations.
- Consideration of bias factors: by considering bias factors (e.g., by including multiple measurements), one can ensure that the results of the study are not due to chance or measurement error.
There are other ways to support or challenge the external validity of a study.
Frequently asked questions and answers: Validity
What are the biggest errors in validity?
There are several factors that can affect the validity of a statistic. Some possible errors that can occur in validity are:
– Bias due to selective sampling: If the sample used for the study is not representative of the population under consideration, this can lead to bias in the results.
– Measurement error bias: If the measurement instrument or method used to collect the data is not reliable or accurate, this can lead to bias in the results.
– Bias due to experimental interference: If the independent variable in an experimental study is not controlled, this can lead to bias in the results.
– Bias due to bias factors: If bias factors that could affect the results of the study are not taken into account, this can lead to bias in the results.
There are other factors that can affect the validity of a statistic. It is important to consider the specific requirements of the study and the variables under consideration to adequately support or challenge the validity of a statistic.
What is an example of high validity?
An example of high validity would be when a measurement instrument or method actually measures what you want to measure and does not present biases or approximations.
For example, an example of a measurement tool with high validity would be a blood pressure monitor that accurately measures blood pressure and presents no bias. An example of a method with high validity would be, for example, a survey that is representative of the population under consideration and presents no bias.
It is important to note that there is no absolute “right” or “wrong” method to support or question the validity of a statistic. Rather, which methods are best suited to support or challenge the validity of a statistic depends on the specific circumstances and variables under consideration. However, there are some general requirements that apply to supporting validity, such as:
– Use of representative samples: By using representative samples, one can ensure that the results of the study are generalizable to other populations.
– Consideration of bias factors: By considering bias factors (e.g., by including multiple measurements), one can ensure that the results of the study are not due to chance or measurement error.
There are other ways to support or challenge the validity of a statistic. It is important to consider the specific requirements of the study and the variables under consideration to adequately support or challenge the validity of a statistic.
What is the difference between validity and reliability?
Validity and reliability are two important concepts in statistics that are often confused with each other. The difference between validity and reliability can be summarized as follows:
– Validity: validity refers to whether a measurement tool or method actually measures what you want to measure. A statistic is valid if it actually measures what you want to measure and is not just an approximation or a distortion of it.
– Reliability: Reliability refers to how reliable or stable a measurement tool or method is. A statistic is reliable if it produces similar results when used repeatedly.
It is important to note that validity and reliability are two separate concepts that are not necessarily related. For example, a measurement instrument or method may be valid but not reliable, or vice versa. It is therefore important to consider both the validity and reliability of a statistic to ensure that the results are valid and reliable.
What are the types of validity?
There are different types of validity, which can be defined differently depending on the specific context and variables under consideration. Here are some examples of types of validity:
Construct validity: construct validity refers to whether a measurement procedure or analysis actually measures what it intends to measure. For example, one might question the construct validity of a test measuring intelligence if the test also measures other factors such as educational attainment or personality.
Content-related validity: content-related validity refers to whether a measurement procedure or analysis does justice to the most important aspects of a construct (e.g., a trait or ability). For example, one might question the content-related validity of a test measuring leadership competencies if the test depicts only a fraction of the skills that are important to leadership competencies.
Criterion-related validity: criterion-related validity refers to whether a measurement procedure or analysis makes predictions about a particular criterion (e.g., school performance, job performance, etc.). There are two types of criterion-related validity: concurrent-related validity and predictive-related validity. Concurrent-related validity refers to whether the measurement procedure or analysis agrees well with other measurement procedures or analyses that measure the same criterion. Predictive-related validity refers to whether the measurement procedure or analysis predicts well how the criterion will develop in the future.
There are other types of validity, not all of which are listed here. It is important to note that the validity of a measurement procedure or analysis can be defined differently depending on the specific context and variables under consideration.
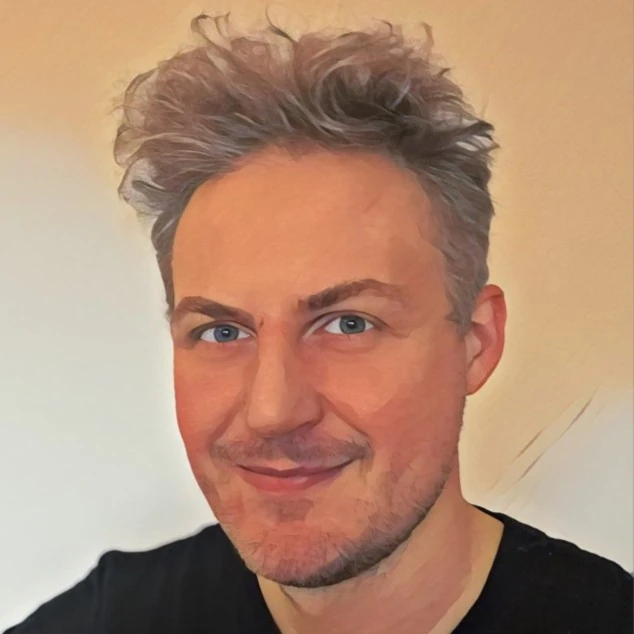
About me: Dr. Peter Merdian
Expert for Neuromarketing, Statistics and Data Science
Hi, I’m Peter Merdian and Statistic Hero is my heart project to help people get started with statistics easily. I hope you like the tutorials and find useful information! I myself have a PhD in Neuromarketing and love data-driven analysis. Especially with complex numbers. I know from my own experience all the problems you have as a student in your studies. That’s why the instructions are as practical and simple as possible. Feel free to use the instructions with your own data sets and calculate exciting results. I wish you success in your studies, research or work. Want to give me feedback or reach me? Dr. Peter Merdian LInkedIn